Plane Poiseuille flow - BVP
August 11, 2011 at 04:49 PM | categories: odes | View Comments
Plane Poiseuille flow - BVP
in the pressure driven flow of a fluid with viscosity between two stationary plates separated by distance
and driven by a pressure drop
, the governing equations on the velocity
of the fluid are (assuming flow in the x-direction with the velocity varying only in the y-direction):
with boundary conditions and
, i.e. the no-slip condition at the edges of the plate.
we convert this second order BVP to a system of ODEs by letting ,
and then
. This leads to:
with boundary conditions and
.
for this problem we let the plate separation be d=0.1, the viscosity , and
.
function main
clc; close all; clear all; d = 0.1; % plate thickness
we start by defining an initial grid to get the solution on
x = linspace(0,d);
Next, we use the bvpinit command to provide an initial guess using the guess function defined below
solinit = bvpinit(x,@guess);
we also need to define the ode function (see odefun below).
and a boundary condition function (see bcfun below).
now we can get the solution.
sol = bvp5c(@odefun,@bcfun,solinit)
sol = solver: 'bvp5c' x: [1x100 double] y: [2x100 double] idata: [1x1 struct] stats: [1x1 struct]
sol is a Matlab struct with fields. the x field contains the x-values of the solution as a row vector, the y field contains the solutions to the odes. Recall that U1 is the only solution we care about, as it is the original u(x) we were interested in.
y = sol.x; u1 = sol.y(1,:); u2 = sol.y(2,:); plot(y,u1); figure(gcf) xlabel('distance between plates') ylabel('fluid velocity') % the analytical solution to this problem is known, and we can plot it on % top of the numerical solution mu = 1; Pdrop = -100; % this is DeltaP/Deltax u = -(Pdrop)*d^2/2/mu*(y/d-(y/d).^2); hold on plot(y,u,'r--')
As we would expect, the solutions agree, and show that the velocity profile is parabolic, with a maximum in the middle of the two plates. You can also see that the velocity at each boundary is zero, as required by the boundary conditions
function Uinit = guess(x) % this is an initial guess of the solution. We need to provide a guess of % what the function values of u1(y) and u2(y) are for a value of y. In some % cases, this initial guess may affect the ability of the solver to get a % solution. In this example, we guess that u1 and u2 are constants U1 = 0.1; U2 = 0; Uinit = [U1; U2]; % we return a column vector function dUdy = odefun(y,U) % here we define our differential equations u1 = U(1); u2 = U(2); mu = 1; Pdrop = -100; % this is DeltaP/Deltax du1dy = u2; du2dy = 1/mu*Pdrop; dUdy = [du1dy; du2dy]; function res = bcfun(U_0,U_d) % this function defines the boundary conditions for each ode. % we define the velocity at y=0 and y=d to be zero here. If the plates are % moving, you would substitute the velocity of each plate for the zeros. res = [0 - U_0(1); 0 - U_d(1)]; % categories: ODEs % tags: math, fluids
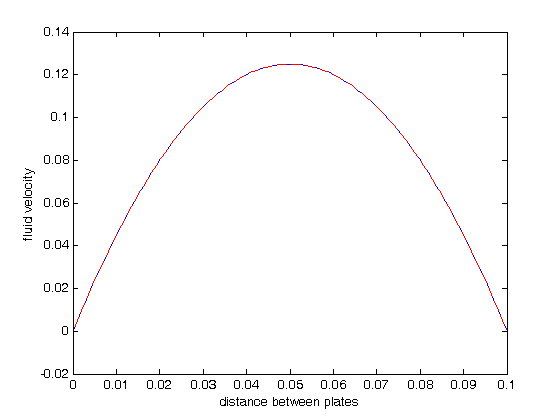